

There may be ways to mine more material that can be fed into the model. Read: What happens when AI has read everything? What this means, the University of Alberta computer scientist Rich Sutton told me, is that language models are only “weakly scalable.” (Computation power, too, could become a limiting factor, though most researchers find this prospect less concerning.) Their first objections are practical: The bigger a language model gets, the more data are required to train it, and we may well run out of high-quality, published text that can be fed into the model long before we achieve anything close to what the maximalists envision. To those in the scaling-skeptical camp, the maximalist stance is magical thinking. His sympathies lie with the opposite side in the debate. “I can see how one might look at that and think, Okay, if that’s the case, maybe we can just keep scaling indefinitely and we’ll clear all the remaining hurdles on the path to human-level intelligence,” Millière said. Alex Dimakis, a computer scientist at the University of Texas at Austin and a co-director of the Institute for Foundations of Machine Learning, told me he became “much more of a scaling maximalist” after seeing all the ways in which GPT-3 has surpassed earlier models. Supersized models have gained the sudden ability to do triple-digit arithmetic, detect logical fallacies, understand high-school microeconomics, and read Farsi. “The Overton window has shifted among AI researchers.” And not without reason: Scaling, AI researchers have found, not only hones abilities that language models already possess-making conversations more natural, for example-but also, seemingly out of nowhere, unlocks new ones. “This once was a view that would have been considered perhaps ludicrous or at least wildly optimistic,” he said. The notion that simply inflating a model will endow it with fundamentally new abilities might seem prima facie ridiculous, and even a few years ago, Millière told me, experts pretty much agreed that it was. Nando de Freitas, the research director at DeepMind, epitomized the position last year when he tweeted, “It’s all about scale now! The Game is Over!” (He did go on, confusingly, to enumerate several other ways he thinks models must improve DeepMind declined to make de Freitas available for an interview.)
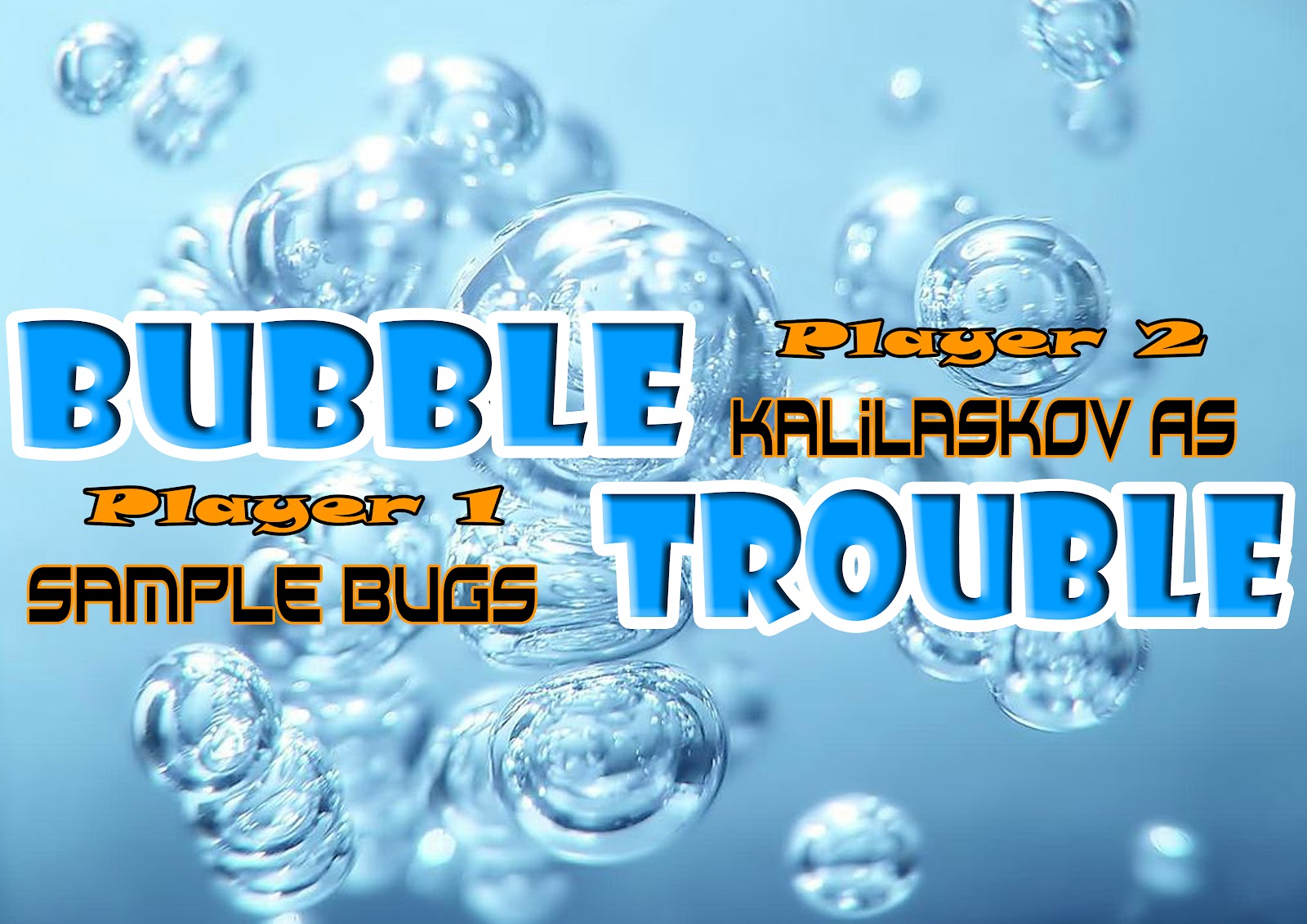
Their basic idea is that the structure of existing technologies will be sufficient to produce AI with true intelligence (whatever you interpret that to mean) all that’s needed at this point is to make that structure bigger-by multiplying the number of parameters and shoveling in more and more data. Raphaël Millière, a Columbia University philosopher whose work focuses on AI and cognitive science, coined the term to refer to the group most bullish about the transformative potential of ramping up. On one side, you have the so-called scaling maximalists. This turns out to be the subject of intense debate among the experts. Will the technology continue to improve indefinitely as more and more data are crammed into its maw? When it comes to AI, how much does size matter? Implicit in the illustration (or at least in the way people seem to have interpreted it) is the assumption that more parameters-which is to say, more knobs that can be adjusted during the learning process in order to fine-tune the model’s output-always lead to more intelligence. Wherever the 100-trillion-parameter rumor originated, OpenAI’s CEO, Sam Altman, has said that it’s “ complete bullshit.” Another problem is that it elides a deeper and ultimately far more consequential question for the future of AI research.
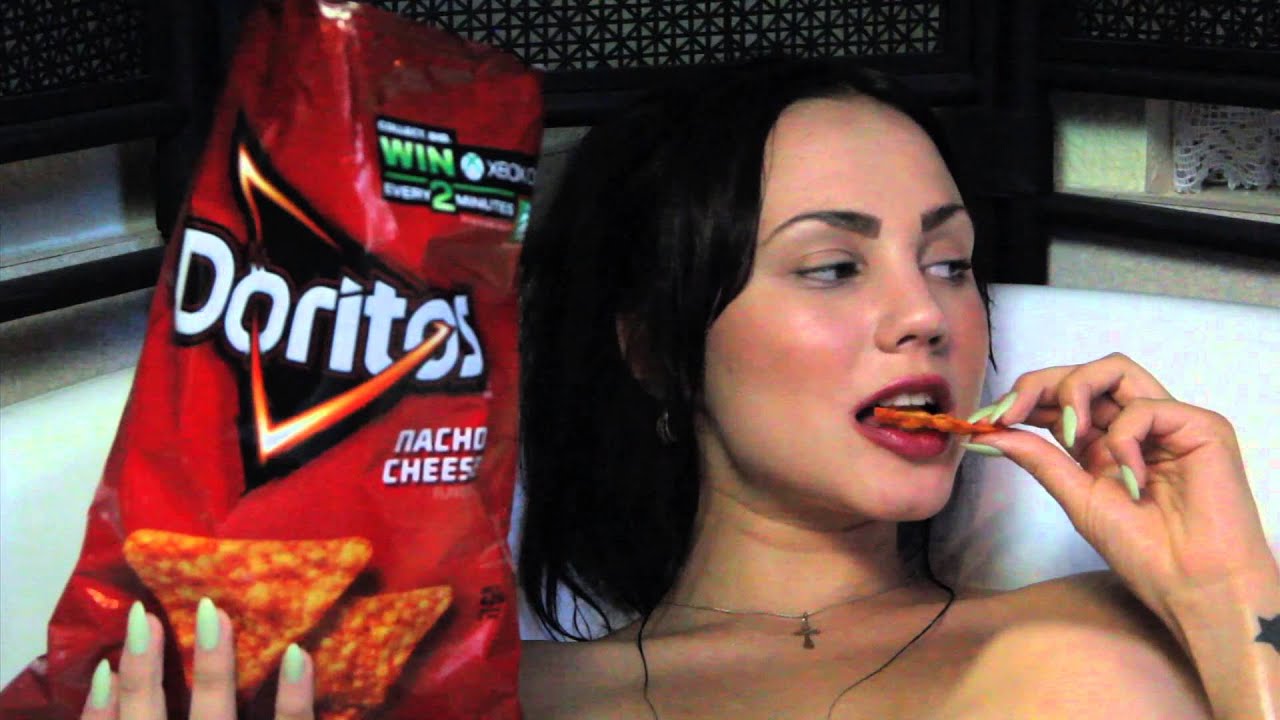
One problem with this hype is that it’s factually inaccurate. The new model, one evangelist tweeted, “will make ChatGPT look like a toy.” “Buckle up,” tweeted another. An illustration shows a tiny dot representing GPT-3 and its “175 billion parameters.” Next to it is a much, much larger circle representing GPT-4, with 100 trillion parameters. One post that has circulated widely online purports to evince its extraordinary power.
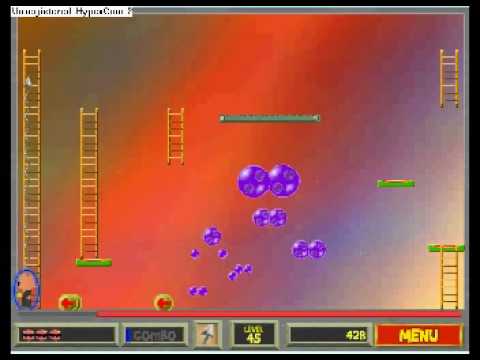
OpenAI’s GPT-4 language model-much anticipated yet to be released-has been the subject of unchecked, preposterous speculation in recent months. As a rule, hyping something that doesn’t yet exist is a lot easier than hyping something that does.
